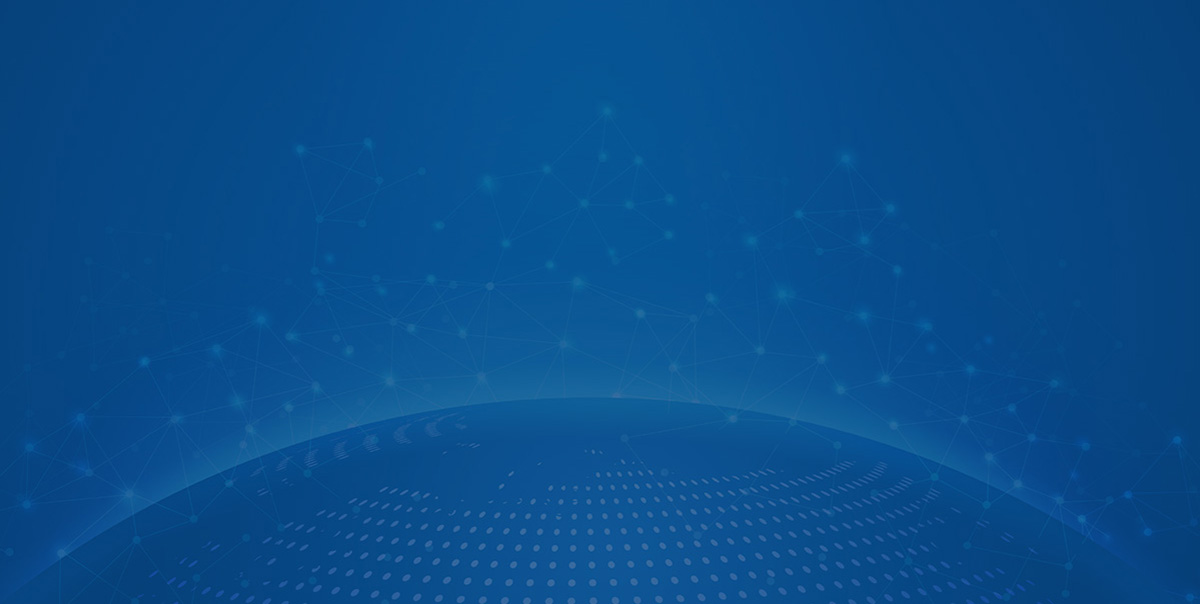
Real-time interaction between programmed processes and included algorithms together with clinical users can create knowledge by:
- presenting analytic results for clinician selection/verification
- recording selections for subsequent application/optimization
- notifying clinicians of pre-defined event occurrence and prompting for next action, free-form or from defined options
- accumulating decisions and results for “rounding” review, verification and new knowledge accrual for reapplication
“Consider the challenge of reading electrocardiograms. Doctors look for a handful of features to diagnose ischemia or rhythm disturbances — but can we ever truly “read” the waveforms in a 10-second tracing, let alone the multiple-day recording of a Holter monitor? Algorithms, by contrast, can systematically analyze every heartbeat. There are early signs that such analyses can identify subtle microscopic variations linked to sudden cardiac death.3 If validated, such algorithms could help us identify and treat the tens of thousands of Americans who might otherwise drop dead unexpectedly in any given year. And they could guide basic research on the mechanisms of newly discovered predictors.
Algorithms have also been deployed for an analysis of massive amounts of EHR data whose results suggest that type 2 diabetes has three subtypes, each with its own biologic signature and disease trajectory.4 Knowing which type of patients we’re dealing with can help us deliver treatments to those who benefit most and may help us understand why some patients have complications and others don’t.
There is little doubt that algorithms will transform the thinking underlying medicine. The only question is whether this transformation will be driven by forces from within or outside the field. If medicine wishes to stay in control of its own future, physicians will not only have to embrace algorithms, they will also have to excel at developing and evaluating them, bringing machine-learning methods into the medical domain.
Machine learning has already spurred innovation in fields ranging from astrophysics to ecology. In these disciplines, the expert advice of computer scientists is sought when cutting-edge algorithms are needed for thorny problems, but experts in the field — astrophysicists or ecologists — set the research agenda and lead the day-to-day business of applying machine learning to relevant data.
In medicine, by contrast, clinical records are considered treasure troves of data for researchers from nonclinical disciplines. Physicians are not needed to enroll patients — so they’re consulted only occasionally, perhaps to suggest an interesting outcome to predict. They are far from the intellectual center of the work and rarely engage meaningfully in thinking about how algorithms are developed or what would happen if they were applied clinically.
But ignoring clinical thinking is dangerous. Imagine a highly accurate algorithm that uses EHR data to predict which emergency department patients are at high risk for stroke. It would learn to diagnose stroke by churning through large sets of routinely collected data. Critically, all these data are the product of human decisions: a patient’s decision to seek care, a doctor’s decision to order a test, a diagnostician’s decision to call the condition a stroke. Thus, rather than predicting the biologic phenomenon of cerebral ischemia, the algorithm would predict the chain of human decisions leading to the coding of stroke.”